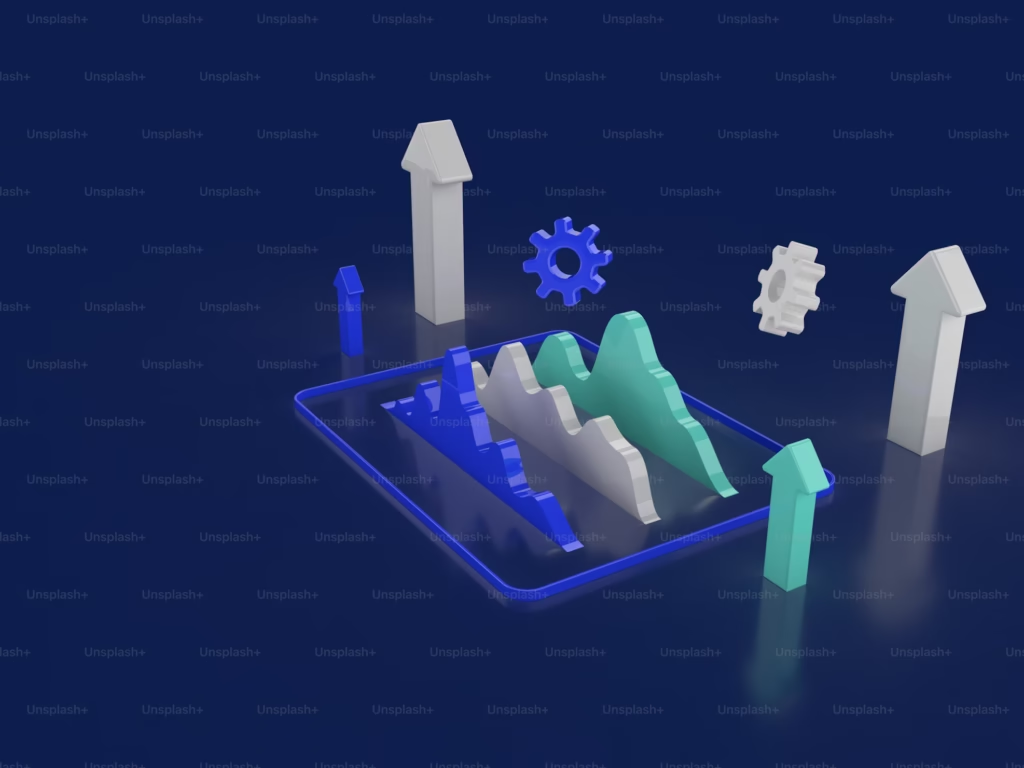
Data modeling is the foundation of an efficient data warehouse. Structuring data to meet analytical requirements ensures streamlined processing, minimizes redundancy, and supports better decision-making. This guide explores how data modeling enhances data warehouses, offering practical tips and examples.
What is Data Modeling?
Data modeling is the process of organizing and structuring data in a system to meet user requirements effectively. It involves:
- Identifying the types of data to be stored.
- Defining relationships between datasets.
- Incorporating rules and constraints to ensure data integrity and consistency.
The ultimate goal of data modeling is to cater to user needs efficiently while maintaining a robust and optimized system structure.
Why is Data Modeling Critical for Data Warehouses?
Data modeling for data warehouses focuses on organizing data to enable swift analysis and reporting. Unlike transactional databases, data warehouses prioritize efficient querying and aggregations for business intelligence tasks.
Key Approaches to Data Modeling
- Star Schema (Simplified for Fast Queries)
- Centralized fact table connected to multiple dimension tables.
- Example:
- Fact Table: Sales (e.g., Sales ID, Amount, Date).
- Dimension Tables: Product, Customer, Time.
- Snowflake Schema (Normalized for Storage Optimization)
- Extension of the star schema with further normalization of dimensions.
- Example: Product dimension split into Product, Category, and Supplier tables.
- Hybrid Approaches
- Combines star and snowflake schemas for flexibility based on data size and usage.
Best Practices in Data Modeling for Data Warehouses
- Understand Business Goals: Align the model with specific reporting and analytics needs.
- Optimize for Querying: Ensure dimension tables are denormalized for faster lookups.
- Maintain Consistency: Use surrogate keys for uniform data representation.
For a detailed overview of schema design, check out Understanding Star and Snowflake Schemas.
Benefits of Data Modeling in Data Warehousing
Enhanced Performance
Proper data models ensure optimized query execution, saving time and resources.
Scalability and Flexibility
Models can adapt to growing datasets and changing business needs without disrupting operations.
For more insights, explore The Basics of Database Design.
Types of Data Modeling Based on User Needs
Data modeling varies depending on the system type and the end user. Let’s break this down further:
1. Type of System
- Online Transactional Processing (OLTP):
OLTP systems focus on frequent, real-time transactions. Databases with normalized structures are ideal for these systems as they minimize redundancy and optimize storage. - Online Analytical Processing (OLAP):
OLAP systems are used for analysis and reporting. Denormalized structures, such as star or snowflake schemas, are preferred as they provide quick access to aggregated data.
2. Type of Consumer
- Data Analysts:
Analysts need simpler table structures for easy querying. Redundant data may be acceptable if it speeds up access. - Data Engineers:
Engineers prioritize optimized and complex structures. They are skilled at handling intricate queries and working with normalized datasets.
Data modeling is essential for creating effective data warehouses. Whether using a star schema for simplicity or a snowflake schema for optimization, aligning the model with business goals ensures meaningful analytics. Start applying these principles to design a data warehouse that truly meets your needs.
Example Use-case:
- A student scored 95 marks in Mathematics.
In this case, 95 marks is a FACT.
The answers to the WH questions, such as:
- Who scored the marks? (Student Name)
- Which subject is it? (Mathematics)
- When was the exam conducted? (Date)
- Who was the teacher? (Instructor Name)
These represent the DIMENSIONS.
Key Notes:
- Facts vs Dimensions: Facts (e.g., marks scored) are generally fewer compared to dimensions (e.g., student, subject, teacher, etc.).
- Relationships:
- A Fact table connects to multiple Dimension tables, but Dimension tables do not connect directly to one another.
- Dimension tables are denormalized for ease of querying and performance optimization.
- Fact Table Characteristics:
- Contains keys referencing Dimension tables.
- Has fewer fact values but connects to many dimensions.
- Grows over time due to transactional nature (e.g., new exam results).
- Dimension Table Characteristics:
- Relatively smaller than the Fact table.
- Contain descriptive data for analysis.
This schema is commonly used in star schema modeling in data warehousing.
Here are some popular data warehousing solutions widely used in the industry:
Cloud-Based Solutions
- Amazon Redshift
- A fully managed data warehouse solution by AWS.
- Supports massive parallel processing (MPP) for high-speed querying.
- Google BigQuery
- A serverless, highly scalable warehouse by Google Cloud.
- Ideal for real-time analytics and large-scale data processing.
- Microsoft Azure Synapse Analytics
- Integrates big data and data warehousing seamlessly.
- Offers advanced analytics and machine learning integration.
- Snowflake
- A cloud-native solution that separates storage and compute for flexibility.
- Known for its scalability and ease of use.
On-Premises Solutions
- Teradata
- Enterprise-grade data warehousing with robust analytics capabilities.
- Often used for large-scale deployments in traditional setups.
- IBM Db2 Warehouse
- Offers in-memory columnar processing for high-speed analytics.
- Suitable for hybrid cloud and on-premises environments.
- Oracle Autonomous Data Warehouse
- Fully automated warehouse by Oracle.
- Simplifies management and offers integrated analytics.
Open-Source Solutions
- Apache Hive
- Built on Hadoop for big data warehousing.
- Supports SQL-like queries for distributed data processing.
- ClickHouse
- A columnar database management system optimized for analytics.
- Open-source and highly performant.
- Greenplum
- Open-source, MPP-based data platform.
- Ideal for large-scale analytics and machine learning tasks.
These solutions cater to diverse business needs, from small startups to enterprise-scale organizations. The choice depends on factors like scalability, cost, performance, and integration requirements.