In today’s data-driven world, organizations are generating and processing vast amounts of data. Efficiently transforming this data into actionable insights is crucial for success. Apache Spark, an open-source distributed computing system, has emerged as a game-changer in this domain. With its speed, scalability, and versatile APIs, Spark is revolutionizing the way we handle data transformation.
What is Apache Spark?
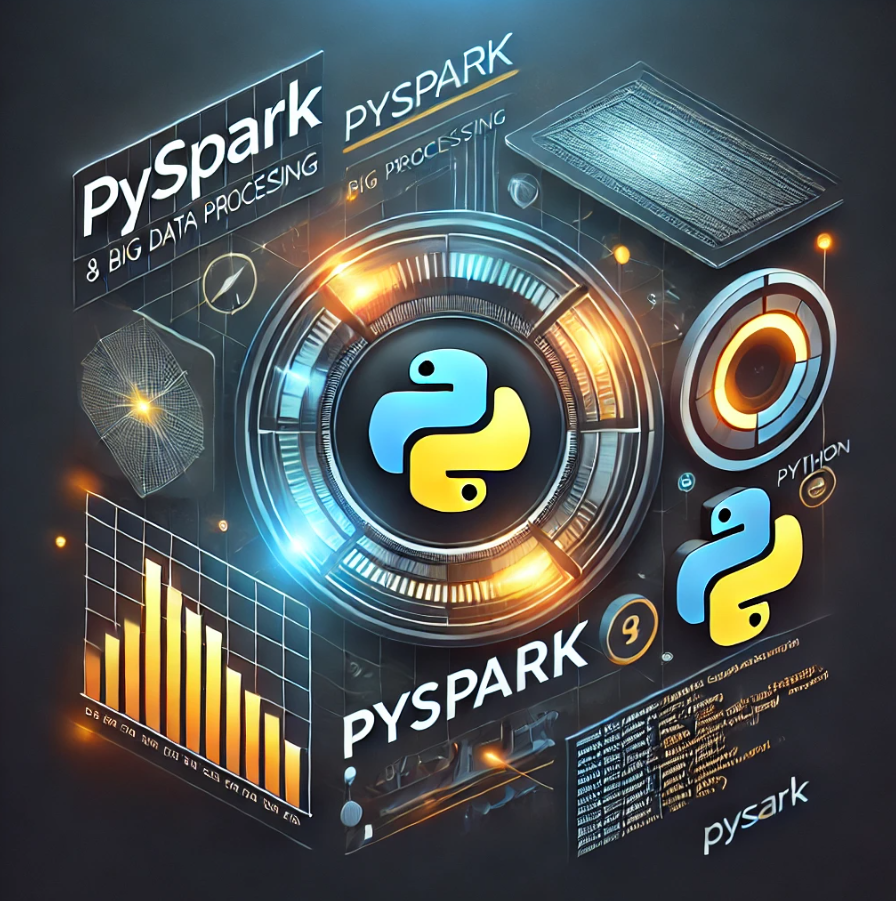
Apache Spark is a unified analytics engine designed for large-scale data processing. It supports multiple programming languages, including Python, Java, Scala, and R, making it accessible to a wide range of developers. Spark’s in-memory processing capabilities allow it to perform tasks up to 100 times faster than traditional big data frameworks like Hadoop MapReduce.
Why Choose Apache Spark for Data Transformation?
- Speed and Efficiency: Spark’s in-memory computation reduces the need for frequent disk I/O, significantly speeding up data transformation tasks.
- Scalability: Designed to handle massive datasets, Spark can seamlessly scale across hundreds or thousands of nodes in a cluster.
- Rich Ecosystem: Spark provides libraries like Spark SQL, MLlib, GraphX, and Structured Streaming, which extend its functionality to include SQL querying, machine learning, graph processing, and real-time data streaming.
- Ease of Use: Its APIs are intuitive and allow developers to express complex transformations in a few lines of code.
Key Features for Data Transformation
- Resilient Distributed Datasets (RDDs): The core abstraction in Spark, RDDs, provides fault tolerance and parallelism, enabling efficient data transformation.
- DataFrame and Dataset APIs: These high-level APIs offer structured data manipulation capabilities and optimization through Catalyst, Spark’s query optimizer.
- Lazy Evaluation: Transformations in Spark are executed only when an action is triggered, allowing for optimized execution plans.
- Built-in Functions: Spark includes a rich set of built-in functions for tasks like filtering, aggregating, and joining datasets, simplifying common transformation workflows.
Common Use Cases
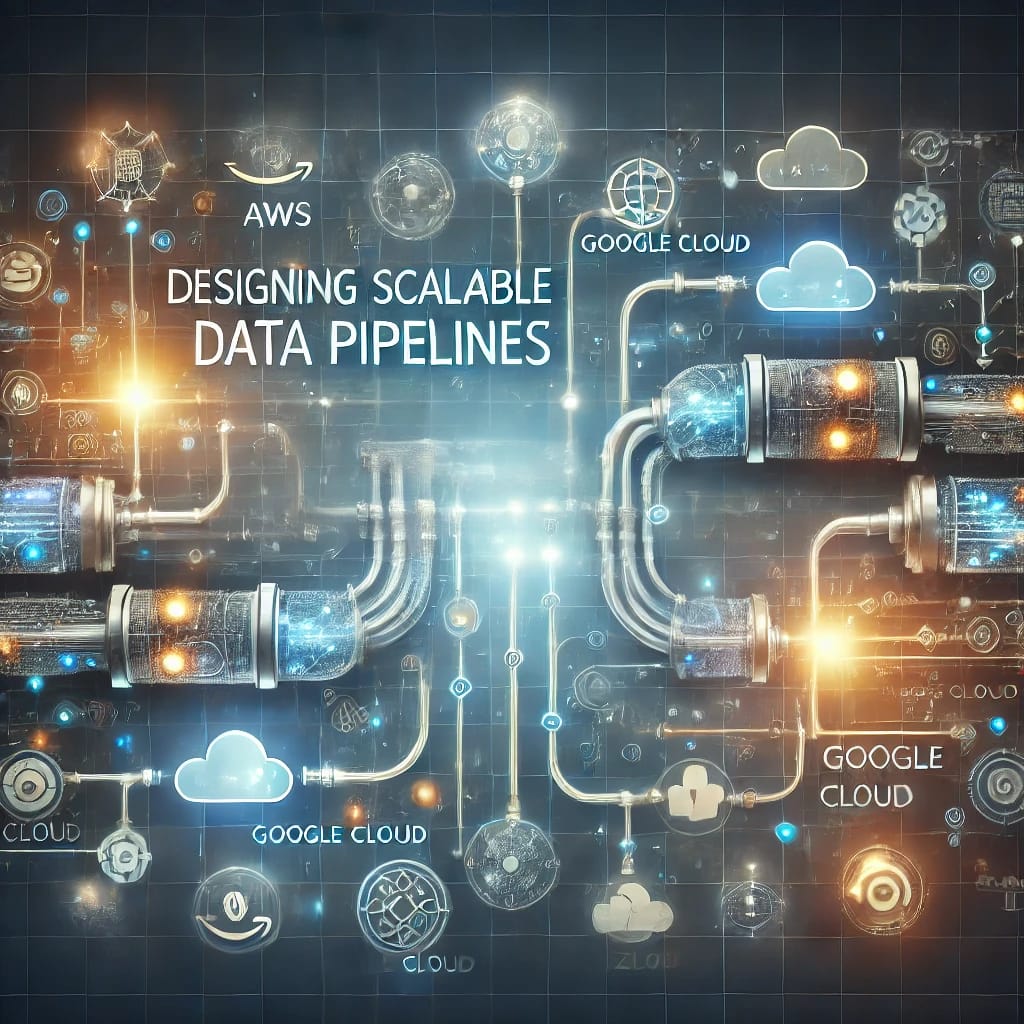
- Data Cleaning: Spark’s ability to handle diverse data sources and formats makes it ideal for cleansing messy datasets.
- ETL Pipelines: Extract, transform, and load operations are streamlined with Spark’s integration capabilities and high-speed processing.
- Feature Engineering: For machine learning, Spark helps preprocess and transform data efficiently, supporting tasks like encoding, scaling, and dimensionality reduction.
- Real-Time Data Processing: With Structured Streaming, Spark enables real-time transformations on data streams, making it perfect for time-sensitive applications.
Getting Started with Apache Spark
To start using Apache Spark for data transformation:
- Set Up Spark: Install Spark on your local machine or use cloud-based services like AWS EMR, Databricks, or Google Dataproc.
- Load Data: Use Spark’s APIs to read data from diverse sources like HDFS, S3, or relational databases.
- Transform Data: Apply a series of transformations using RDDs, DataFrames, or Datasets.
- Persist Results: Save the transformed data to your desired storage system.
Apache Spark stands out as a powerful tool for data transformation, offering speed, flexibility, and scalability. Whether you’re a data engineer building ETL pipelines or a data scientist preparing datasets for machine learning, Spark’s robust features and intuitive APIs make it an indispensable part of your toolkit.
Are you ready to transform your data workflows with Apache Spark? Start exploring its potential today and unlock new insights from your data! read the blogpost.
Pingback: Spark Joins: A Comprehensive Guide - WCBlog