Data joins are a fundamental operation in data analysis and processing. In Spark, they allow you to combine data from two or more DataFrames based on a common key or condition. This blog post will explore different types of joins in Spark, accompanied by code examples and explanations to enhance understanding.
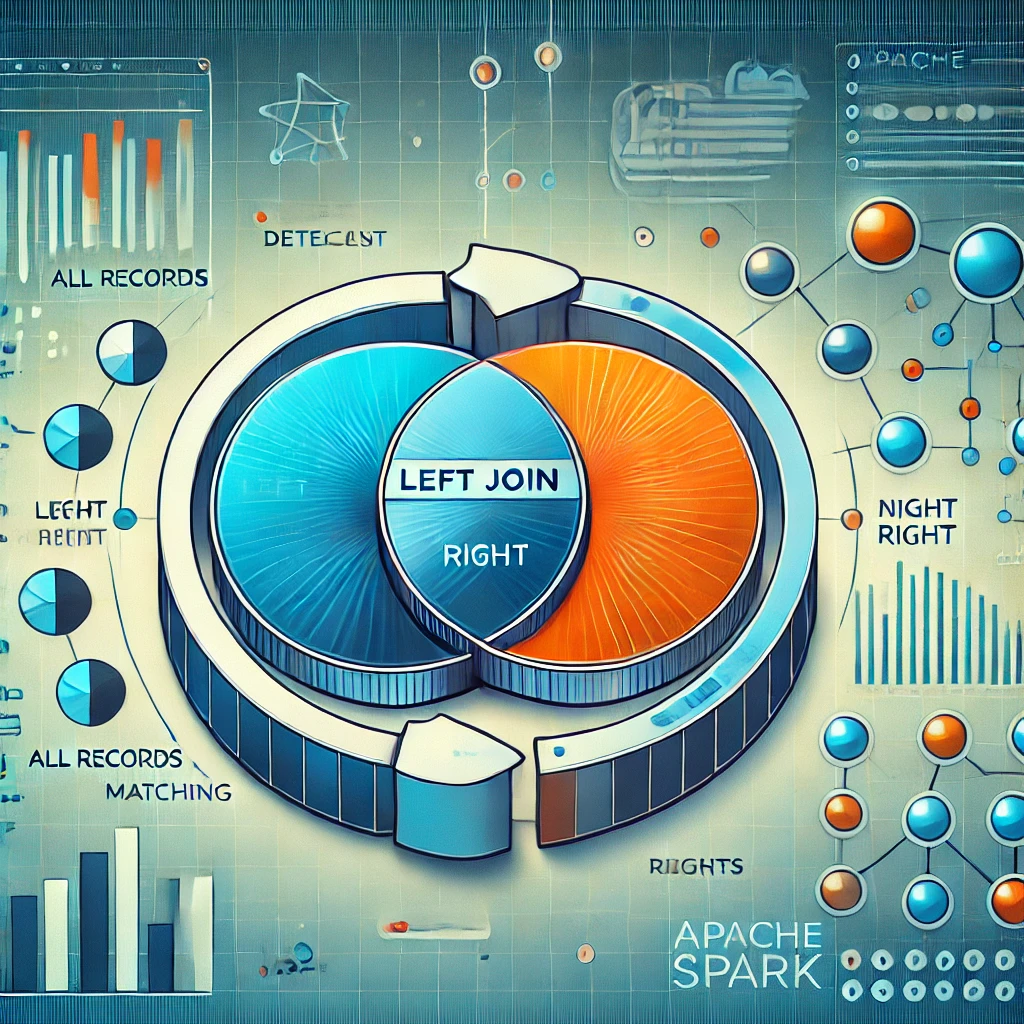
Types of Joins in Spark
The image you shared illustrates the four primary join types in Spark:
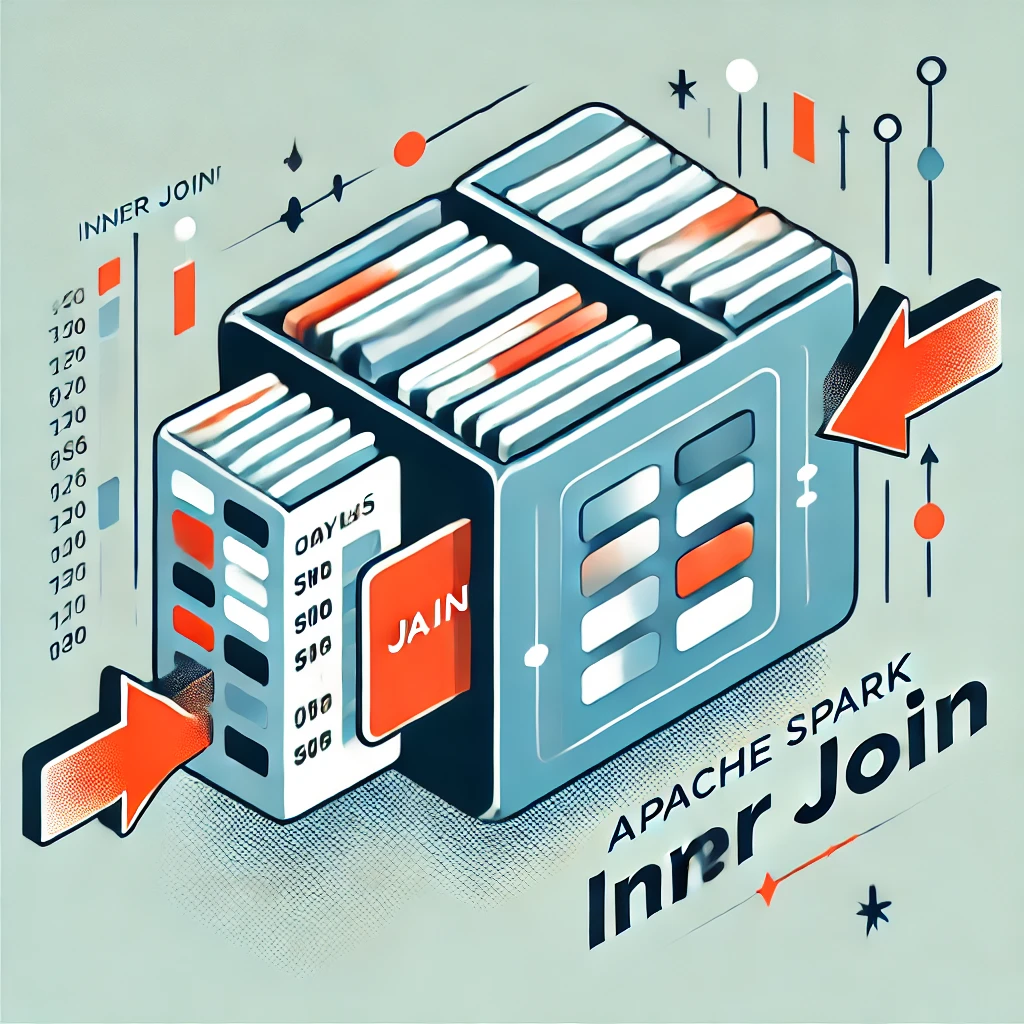
- Inner Join:
- Description: Returns rows only when there is a match in both DataFrames based on the join condition.
- Example:
df1 = spark.createDataFrame([
(1, "Alice"),
(2, "Bob"),
(3, "Charlie")
], ["id", "name"])
df2 = spark.createDataFrame([
(1, "New York"),
(2, "London"),
(4, "Tokyo")
], ["id", "city"])
inner_join_df = df1.join(df2, "id", "inner")
inner_join_df.show()
Output:
+---+------+----+-------+
| id| name| id| city|
+---+------+----+-------+
| 1| Alice| 1|New York|
| 2| Bob| 2| London|
+---+------+----+-------+
Left Join:
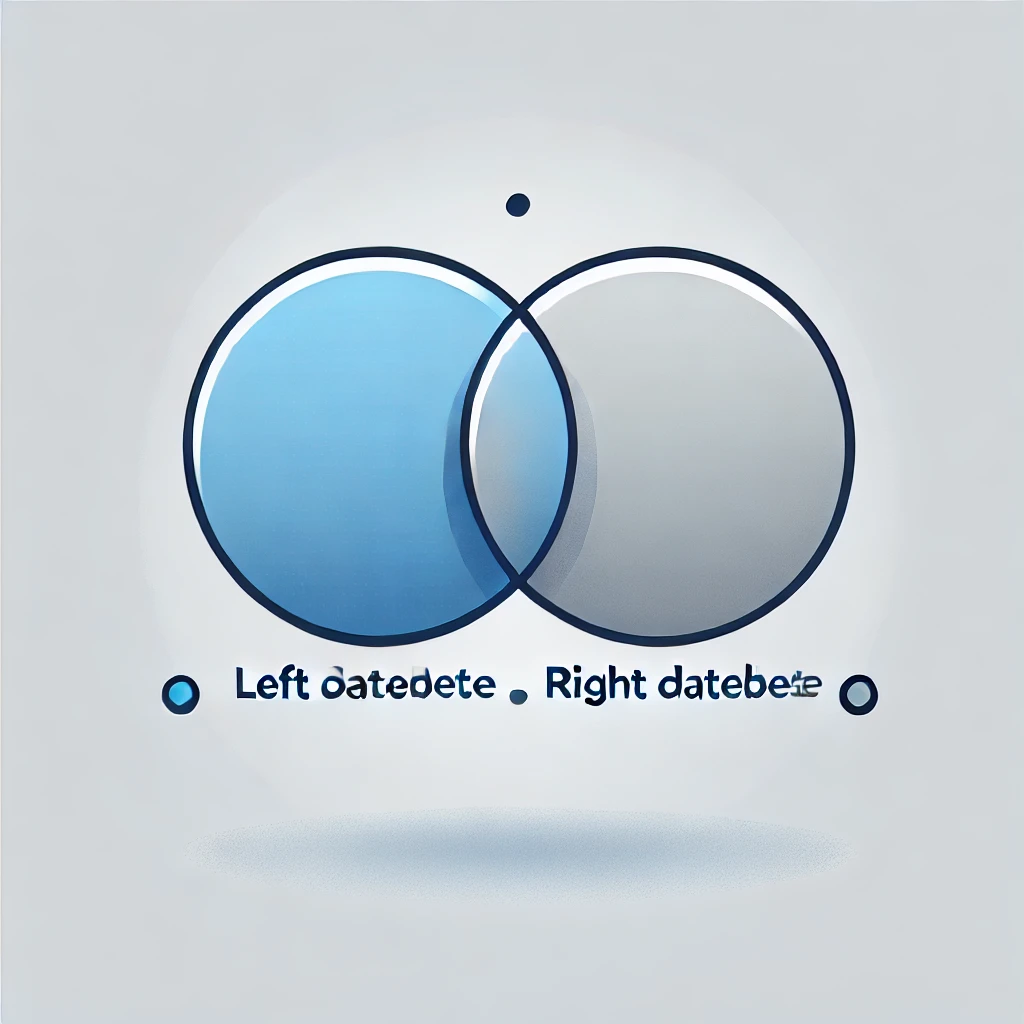
- Description: Returns all rows from the left DataFrame and the matching rows from the right DataFrame. If there is no match in the right DataFrame, null values are filled in.
- Example:
left_join_df = df1.join(df2, "id", "left")
left_join_df.show()
Output:
+---+------+----+-------+
| id| name| id| city|
+---+------+----+-------+
| 1| Alice| 1|New York|
| 2| Bob| 2| London|
| 3|Charlie| null| null|
+---+------+----+-------+
Right Join:
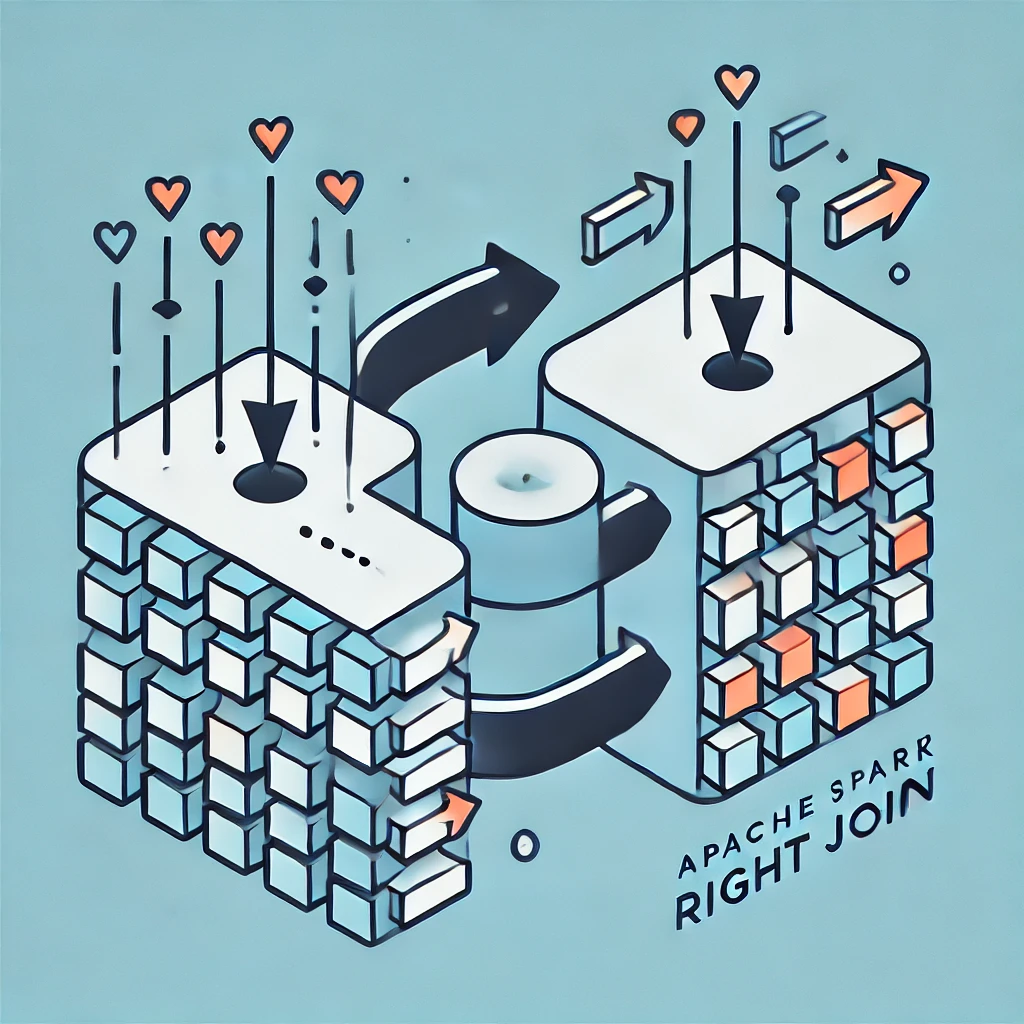
- Description: Returns all rows from the right DataFrame and the matching rows from the left DataFrame. If there is no match in the left DataFrame, null values are filled in.
- Example:
right_join_df = df1.join(df2, "id", "right")
right_join_df.show()
Output:
+----+-------+---+------+
| id| city| id| name|
+----+-------+---+------+
| 1|New York| 1| Alice|
| 2| London| 2| Bob|
| 4| Tokyo| null| null|
+----+-------+---+------+
Full Outer Join:
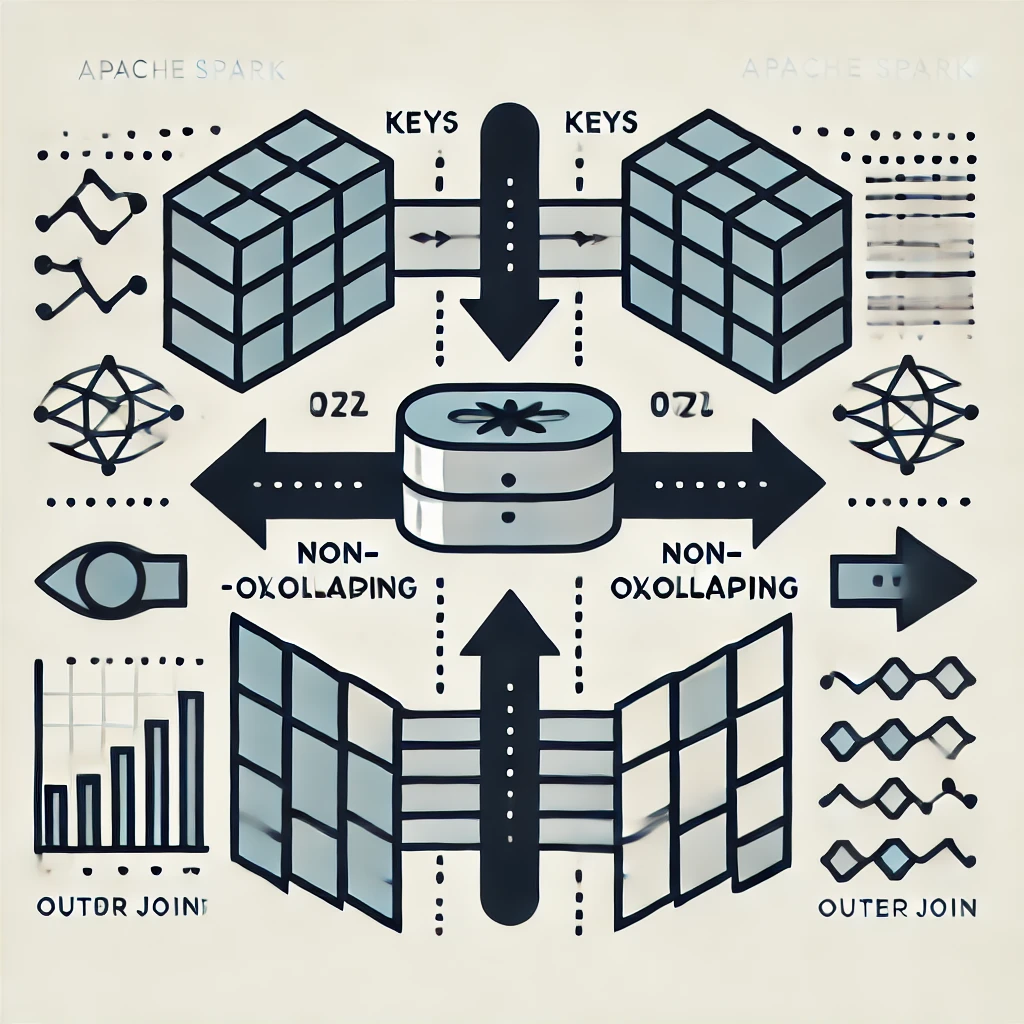
- Description: Returns all rows from both DataFrames, filling in null values for unmatched rows.
- Example:
full_join_df = df1.join(df2, "id", "full")
full_join_df.show()
Output:
+----+------+----+-------+
| id| name| id| city|
+----+------+----+-------+
| 1| Alice| 1|New York|
| 2| Bob| 2| London|
| 3|Charlie| null| null|
| 4| null| 4| Tokyo|
+----+------+----+-------+
Key Points to Remember:
- The join condition can be any expression that evaluates to a boolean value.
- If you don’t specify a join type, Spark defaults to an inner join.
- Joins can be performed on multiple columns using a list of column names.
- For complex join conditions, you can use the
on
clause instead of specifying the join column.
Practical Use Cases:
- Combining data from different sources, such as customer data and order data.
- Enriching data with additional information, such as joining product data with sales data.
- Data cleaning and transformation, such as joining data with reference tables.
You might also like SparkSession and DataFrame Operations