In the realm of data engineering, the choice of tools can significantly impact the efficiency, scalability, and cost-effectiveness of data processing tasks. Recently, Amazon made headlines by transitioning from Apache Spark to Ray for their data lakehouse’s table compaction tasks. This shift not only showcases Ray’s capabilities but also highlights its advantages over traditional frameworks like Spark.
In this blog, we’ll delve into the reasons behind this transition and explore the benefits of utilizing Ray in AWS environments.
The Limitations of Apache Spark
Apache Spark has long been a popular choice for big data processing due to its ability to handle large datasets across distributed computing environments. However, as Amazon’s Business Data Technologies (BDT) team discovered, Spark can struggle under the pressures of extreme scale.
Here are some of the limitations they faced:
- Scalability Challenges: As data volumes increased, Spark’s performance began to degrade. The BDT team experienced missed service level agreements (SLAs) and had to resort to manual tuning of jobs to maintain efficiency.
- Resource Inefficiency: Spark’s high-level abstractions can make it difficult to optimize resource usage effectively, leading to higher operational costs.
- Complexity in Job Management: Managing and scaling Spark jobs often requires significant effort and expertise, making it cumbersome for teams to focus on core tasks.
Why Ray?
Ray is an open-source framework designed for building and running distributed applications. Its architecture allows developers to write applications in a more intuitive way, using Python for distributed computing.
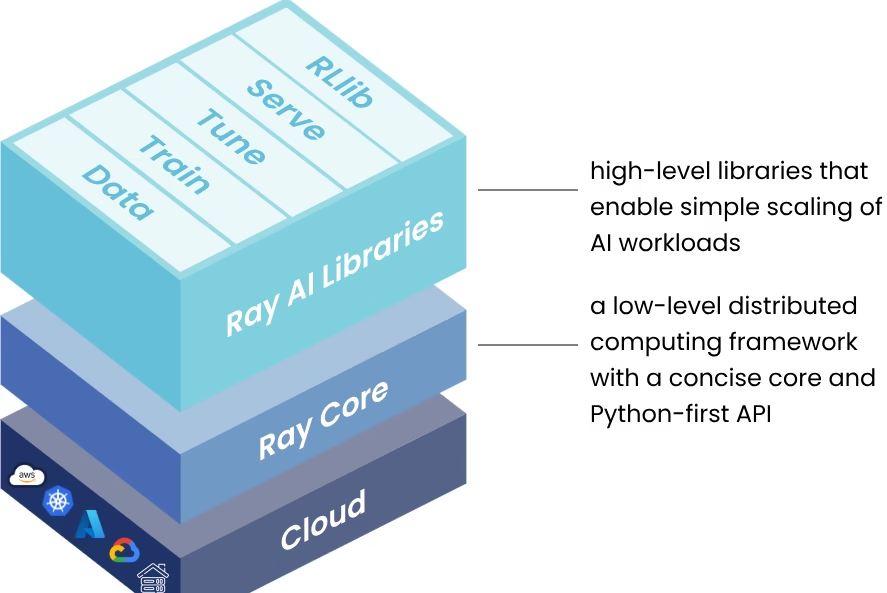
Ray’s unified compute framework consists of three layers:
- Ray AI Libraries–An open-source, Python, domain-specific set of libraries that equip ML engineers, data scientists, and researchers with a scalable and unified toolkit for ML applications.
- Ray Core–An open-source, Python, general purpose, distributed computing library that enables ML engineers and Python developers to scale Python applications and accelerate machine learning workloads.
- Ray Clusters–A set of worker nodes connected to a common Ray head node. Ray clusters can be fixed-size, or they can autoscale up and down according to the resources requested by applications running on the cluster.
Each of Ray’s five native libraries distributes a specific ML task:
- Data: Scalable, framework-agnostic data loading and transformation across training, tuning, and prediction.
- Train: Distributed multi-node and multi-core model training with fault tolerance that integrates with popular training libraries.
- Tune: Scalable hyperparameter tuning to optimize model performance.
- Serve: Scalable and programmable serving to deploy models for online inference, with optional microbatching to improve performance.
- RLlib: Scalable distributed reinforcement learning workloads.
Here’s why Ray became the preferred choice for Amazon:
1. Performance Enhancements
Ray’s ability to handle large-scale data processing was evident during Amazon’s proof-of-concept tests. The results were striking:
- Superior Data Handling: Ray successfully compacted datasets 12 times larger than those managed by Spark, showcasing its capability to process vast amounts of data efficiently.
- Cost Efficiency: By optimizing resource usage, Ray provided a staggering 91% improvement in cost savings and processed 13 times more data per hour compared to Spark.
2. Flexible Programming Model
Ray’s programming model allows developers to define tasks as lightweight functions that can be executed concurrently. This flexibility leads to improved productivity and better control over data processing workflows.
3. Efficient Resource Utilization
Ray’s architecture supports zero-copy object sharing, enabling nodes to share data without incurring the overhead associated with data serialization and deserialization. This results in better performance and reduced memory usage, making it easier to scale applications.
4. Seamless Integration with AWS
Ray’s compatibility with AWS services enhances its appeal for data engineers. Amazon offers various solutions for running Ray, such as AWS Glue for Ray, which allows users to run distributed Python workloads without the need for extensive infrastructure management. This serverless architecture automatically handles scaling and resource allocation, enabling engineers to focus on writing code rather than managing clusters.
Advantages of Using Ray Over Apache Spark
When comparing Ray to Apache Spark, several advantages become apparent:
- Scalability: Ray’s architecture is designed for dynamic scaling, allowing for effortless management of Python workloads across multiple nodes. This is particularly advantageous in environments where data volume fluctuates.
- Cost Savings: The efficiency of Ray translates to significant cost reductions in cloud environments. By minimizing resource wastage, organizations can allocate budgets more effectively.
- Versatility: Ray supports a wide range of workloads, including machine learning model training, hyperparameter tuning, and serving. This versatility makes it a valuable addition to any data engineering toolkit.
- Python-Native API: Ray’s Python-native design allows data engineers to leverage their existing skills and tools, facilitating a smoother transition and faster development cycles.
Whether you are looking to optimize existing data pipelines or explore new data processing opportunities, Ray on AWS is a game-changer worth considering. Its ability to handle diverse workloads with ease positions it as a leading choice for modern data engineering challenges. Embrace the future of data processing with Ray and unlock the full potential of your data infrastructure.
Amazon’s decision to migrate from Apache Spark to Ray illustrates a transformative shift in how large-scale data processing can be approached. For data engineers, Ray presents a compelling alternative that offers enhanced performance, cost savings, and flexibility in managing complex workloads. As the demands on data processing continue to grow, embracing innovative frameworks like Ray can lead to significant improvements in efficiency and effectiveness.
See Blog source aws.